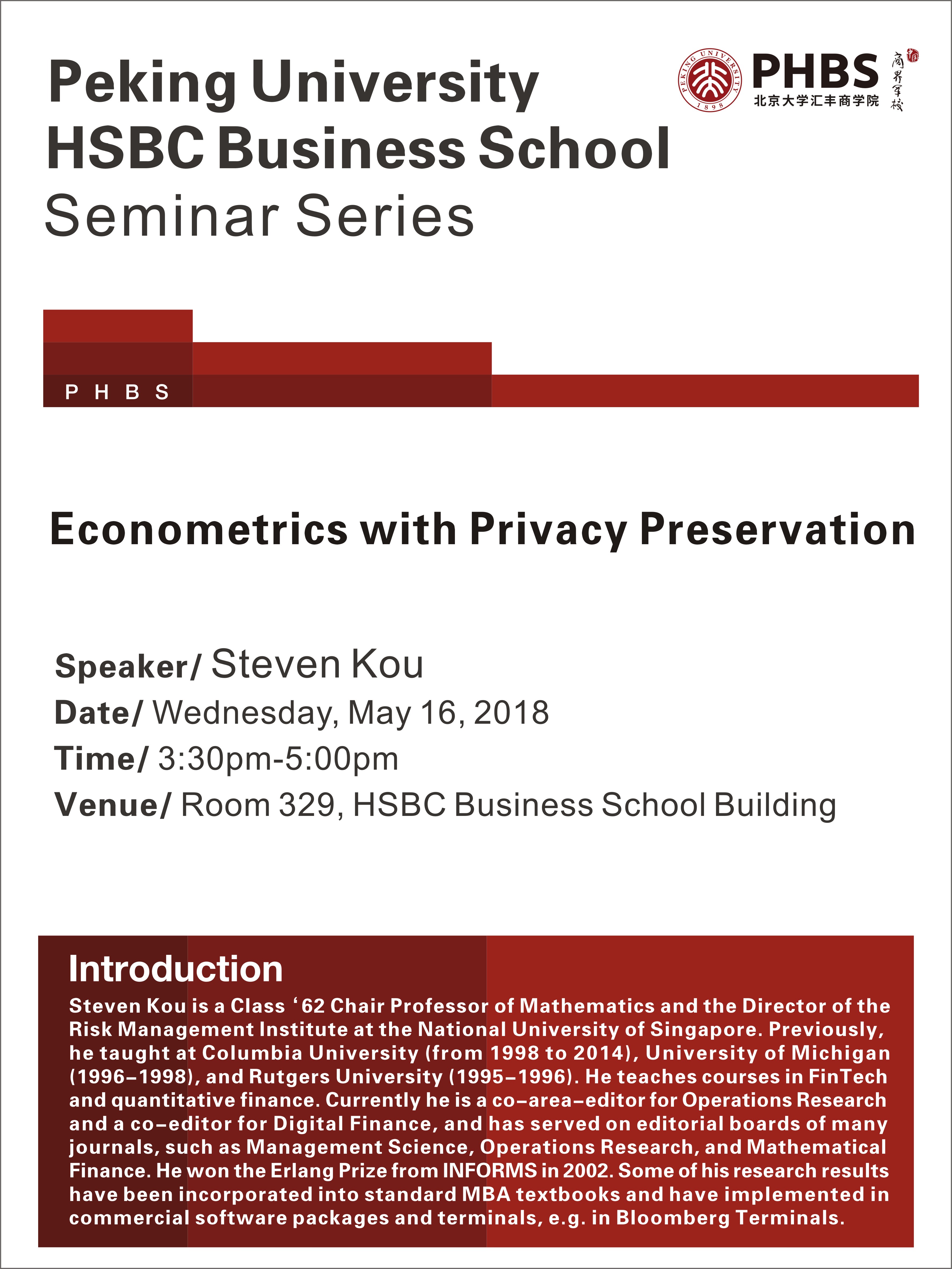
Many data are sensitive in areas such as finance, economics, and other social sciences. We propose an ER (encryption and recovery) algorithm that allows a central administration to do statistical inference based on the encrypted data, while still preserving each party's privacy. Indeed, the central administration can recover just enough information about the original sensitive data so that the required statistical inference can be conducted; furthermore, the algorithm guarantees the privacy for every party involved and works even for a colluding majority in the presence of cyber attack. Applications of our algorithm include linear regression, logistic regression, maximum likelihood estimation, estimation of quantiles, and estimation of empirical distributions. We also illustrate how the ER algorithm can be combined with Fourier transforms as well as how it can be applied to distributed statistical inferences to address the related privacy issue.