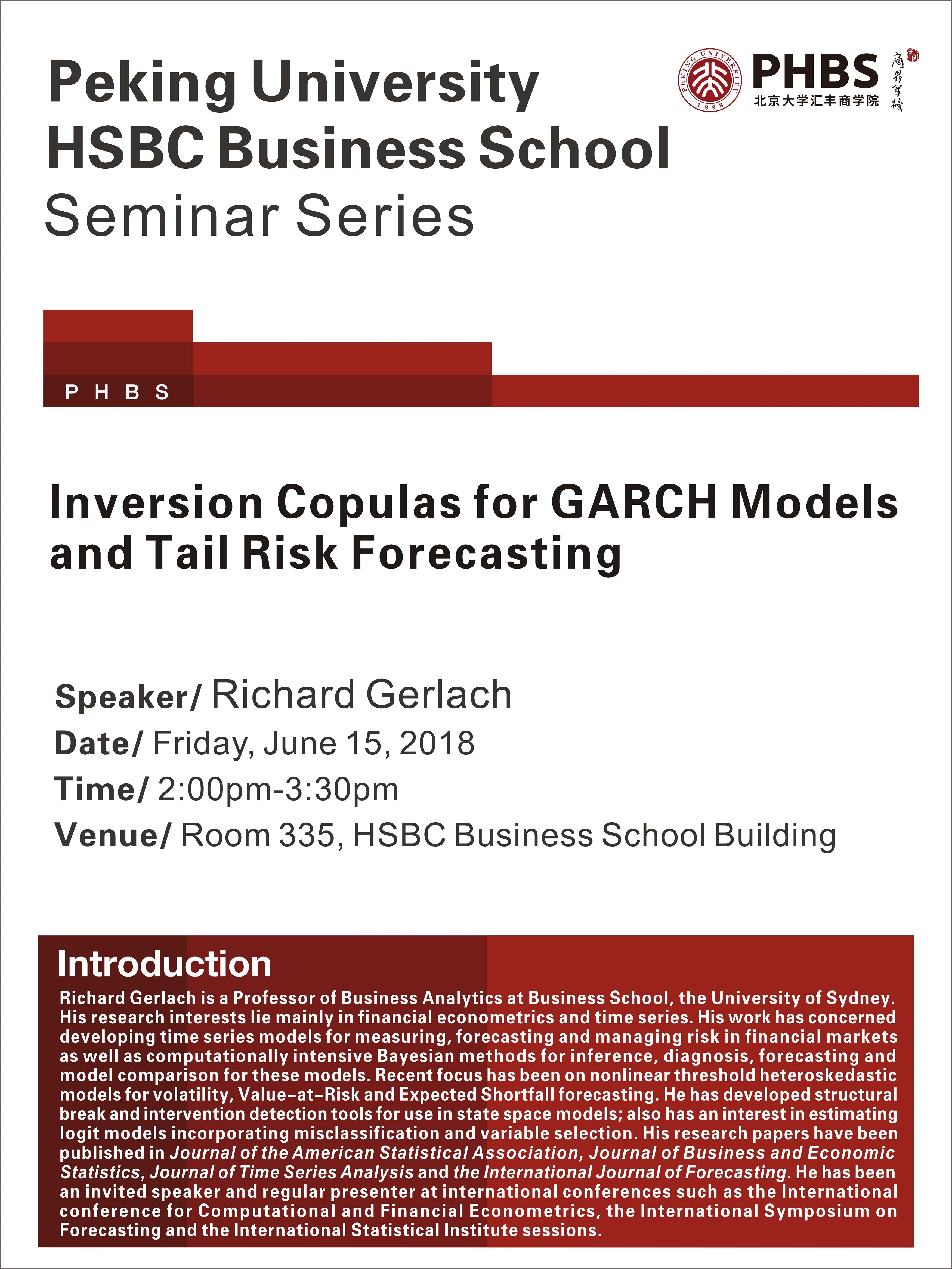
Inversion copulas show promise in modelling latent nonlinear state space models with Markov dependence structures. We extend this idea to cover nonlinear time series with non-Markov dependence, with focus on two special cases: the well-known GARCH and Realized GARCH specifications. Both present challenges in finding and evaluating the implied margin of the latent variable: we discuss some possible solutions here. Likelihood and Bayesian computational methods are derived for estimation, inference and forecasting purposes. The proposed time series inversion copula models are used to model and forecast financial returns from several financial indices, including an emerging markets index and a gold and silver index. The proposed models are competitive for density and tail risk forecasting in these series, compared to a range of popular, competing financial time series models.