The question of which risk factors best explain the cross section of expected equity returns continues to draw attention on account of the large importance of this topic for theoretical and empirical finance. Along with the market factor, hundreds of additional risk factors have emerged, and the set of possible such factors continue to grow. Rather than add to this list, PHBS Assistant Professor Zhao Lingxiao and her coauthors first ask: Could we start with the risk factor collections that have generated support in the recent literature, take the union of the factors in those collections, and then find a new set of risk factors (winners from winners) that gather even more support from the data, on both statistical and financial grounds?
In the paper “Winners from Winners: A Tale of Risk Factors” published in Management Science , they start with the risk factor collections of Fama and French (1993, 2015, 2018), Hou et al. (2015), Stambaugh and Yuan (2017), and Daniel et al. (2020), which gain most support in the literature. In specific, there are 12 factors covering the market, fundamental, aggregated mispricing, and behavioral ones that are widely accepted as important risk factors. In the first part of the analysis, the benchmark model scan and ask what collection of risk factors emerge when each factor is allowed to play the role of a risk factor, that is, an element of the stochastic discount factor (SDF), or a nonrisk factor, to produce different groupings of risk factors in a combinatorial fashion. Each grouping consists of a collection of factors that are risk factors in that grouping, and a complementary collection (the remaining factors) that are nonrisk factors in that grouping.
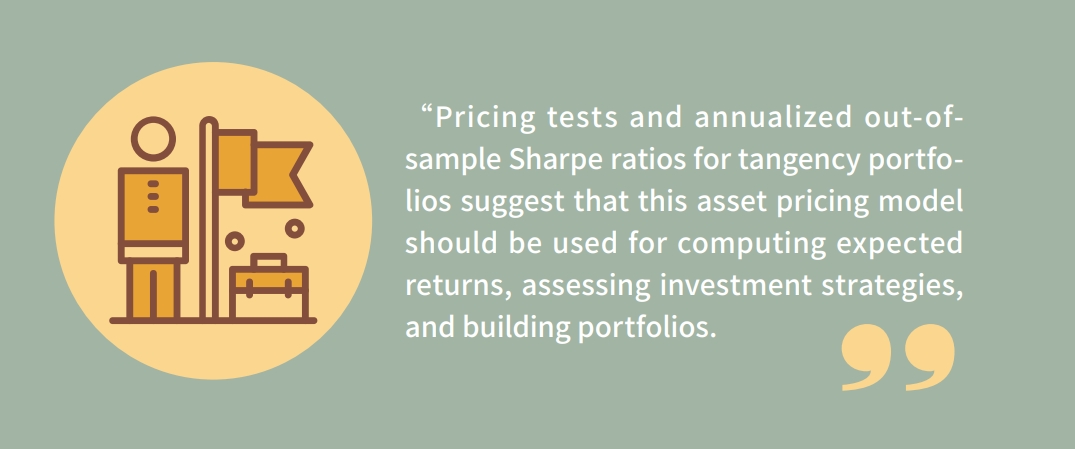
To illustrate, envision a coaching team tasked with assembling a group of athletes for Olympic preparation from a pool of 12 candidates. Taking into account the various events and the associated costs for each athlete, the team must assess the necessity of including each candidate in the group. Consequently, there are a total of 4095 (2^12-1) different combinations for forming this group, considering all possible arrangements.
This benchmark model scan shows that a seven-factor asset pricing model, consisting of {Mkt, SMB, MOM, ROE, MGMT, PERF, and PEAD} gets the most support from the data. This model is closely followed by a second model, a six-factor model that omits PERF, and a third model, a five-factor model, that omits PERF and MOM.
Moreover, to improve the {Mkt, SMB, MOM, ROE, MGMT, PERF, and PEAD} asset pricing model further, they then ask a second question: (2) Can such a benchmark analysis be improved (and by how much) by considering not just the initial factors, but the initial factors plus (say) the 125 anomalies in Green et al. (2017) and Hou et al. (2020)?
To address the question, the authors augment the initial set of factors with the 125 anomalies described above. They take the set of 12 initial factors and find the genuine anomalies, that is, anomalies that cannot be priced by the initial factors, producing 24 genuine anomalies. They then transform the genuine anomalies to principal components (PCs) and consider the first 12 of these PCs as additional factors. Furthermore, they scan (estimate and compare) the 16,777,215 asset pricing models that arise from the 12 initial factors plus the first 12 PC factors. A key feature of this extended model scan is that the initial factors are left as is. Only the genuine anomalies are converted into PCs. A Bayesian comparison of approximately seventeen million models in terms of marginal likelihoods and posterior model probabilities shows that {Mkt, MOM, IA, ROE, MGMT, PERF, PEAD, FIN}, plus the nonconsecutive principal components, {PC1, PC5, PC7} are the best supported risk factors. Pricing tests and annualized out-of-sample Sharpe ratios for tangency portfolios suggest that this asset pricing model should be used for computing expected returns, assessing investment strategies and building portfolios.
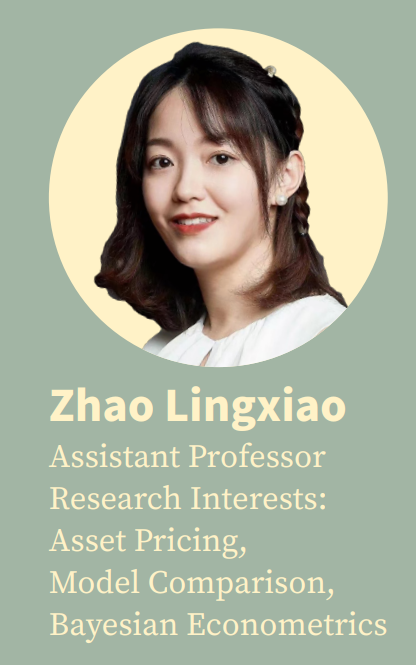