By Zeng Xiaohua
“Big data” has become a ubiquitous term, referring to data that are large and diverse, arriving at a fast rate. Common examples are data from sales, internet searches, social media, and mobile devices. By contrast, marketing managers also talk about “small data” from traditional channels such as surveys, interviews and experiments. Collected after careful research design, they usually contain a relatively small number of observations.
In marketing, big data has transformed business operations. Want to predict when a customer will abandon a service? You can analyze her transactions and predict when she will leave. Want to recommend a product to a customer? You can identify products that share some similarities with items the shopper has purchased or browsed. Strong companies with more customer data (e.g., Amazon and Taobao) may become even stronger by analyzing the data to target customer interests more precisely.
When new business models appear, new data also emerge. When Amazon introduced its cashierless shops (i.e., Amazon Go), people only needed to bring a smartphone to shop and check out by themselves. With new tracking technology, new information may become available, such as the order in which a shopper purchased the products, how long she stayed in front of a shelf, and even items that she examined with interest but put back. These new data can generate deeper insights regarding the consumer decision-making process and product competition. As another example, recently introduced WeChat credit provides consumers a credit rating on e-commerce sites. This scoring system accounts for not only personal records and existing credit histories, but also information such as one’s WeChat friends and their credit [1].
There is no question about the value of big data, but we need to be aware of several pitfalls. The first are the spurious correlations observed in big data. Can you believe that the number of people who drowned by falling into a pool is correlated with the number of films Nicolas Cage appeared in? But this is what the data show [2]. Too often we mix up correlation and causation. The correlation is high between sales of ice cream and swimsuits, but ice cream sales do not lead to swimsuit sales. The cause is clearly hot weather. Big data usually show consumer behavior but seldom illustrate the underlying processes or the cause-and-effect. Sometimes, correlations are helpful for prediction, yet we still need to be careful. For example, the sales of protective masks and video game consoles have been highly correlated during the COVID-19 pandemic, but there is no such correlation without the pandemic. We cannot rely on past correlation to predict the future unless we are sure under what conditions such a relationship holds.
The second pitfall is the biased sample. In big data, we do not sample but only observe what happens. For instance, we want to collect online reviews to infer consumer evaluation of a product. However, there are at least two self-selection biases [3]. It is usually the consumers who
like the product that buy the product and write a product review. Therefore, most reviews are 4- or 5-stars, leading to a flawed conclusion that the product is good. In addition, consumers with extreme opinions (either positive or negative) are more likely to write a review while the majority of consumers remain silent. In one of my projects, I found that reviewers can be very strategic in review writing, perhaps to gain status in the community or to obtain free products. We can use online reviews to determine the perceived product quality only if we can account for these factors.
The third pitfall is related to statistical significance. Given a large amount of data, we can more easily detect a statistically significant result but it may not be economically important. For example, a new advertisement leads to a small increase in consumer sentiment. This small change can turn out to be statistically significant if we analyze a large number of comments, but the magnitude of improvement may be too small to justify the $1 million advertising expenditure. Therefore, we need to pay particular attention to economic significance.
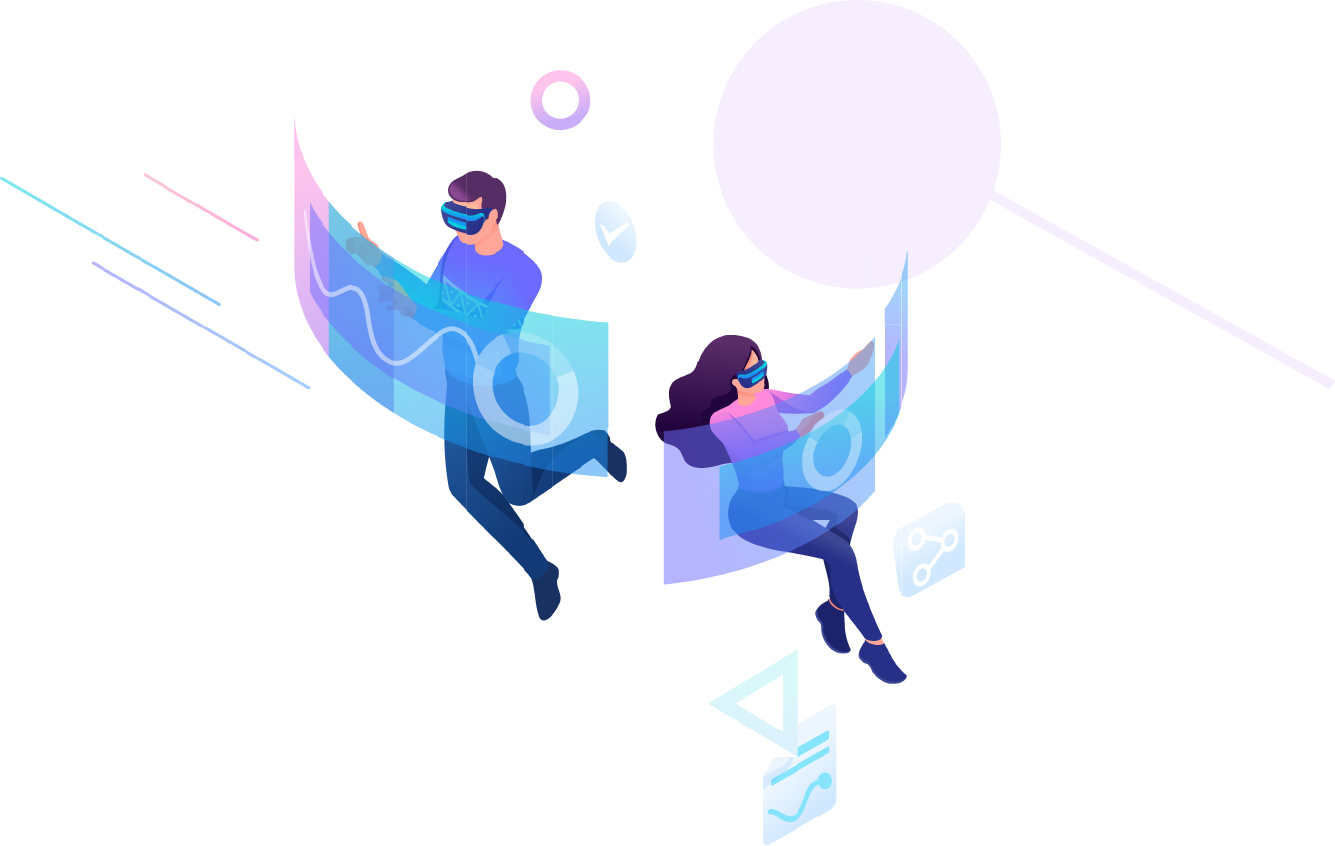
Small data from traditional market research can complement big data in these situations. For example, to understand consumer opinions about a new product, you can conduct a traditional consumer survey and seek a more representative sample. A survey allows you to ask explicit questions. You may not be able to find any online reviews for an unpopular product or for some overlooked product features, but you can directly ask about these in a survey. Yet, two survey bottlenecks are sample size and reporting bias, but these can be solved with appropriate incentive systems. Google introduced a program in 2017 called Google Opinion Rewards, which encourages users to complete surveys and earn credits that can be used for Google Play paid apps. Amazon provides Amazon Mechanical Turk (MTurk), a crowdsourcing marketplace where people complete small tasks, including surveys. It is interesting to see that these two data giants, who are powerful in handling big data, are extending services to the traditional survey market.
To deal with correlation and causation, it is useful to have experiment data. Experiment is a major approach that allows us to infer causal relationships in a strictly controlled environment. For example, Tencent has introduced experiment tools for its advertisers eager to measure whether an advertisement increases sales. It can be challenging to exclude other factors (e.g., competitors, seasonal effects). The tool allows advertisers to divide receivers into two groups, controlling for everything else but the advertisement, and then compare sales with and without the ad.
In fact, many big data companies employ traditional market research methods at the same time. It is common for firms to first conduct a focus group (i.e., interview with a group of consumers) to collect basic insights and then validate the findings with big data. The focus group provides valuable qualitative information while the big data helps quantify the effects.
There are more advanced questions, such as, “Does big data make us more innovative?” Recent research shows that data analytics are complementary to certain types of innovation, but they are not that effective for developing entirely new technologies [4]. To be innovative, we need more than just data.
References:
[1] https://www.thepaper.cn/newsDetail_forward_2838202
[2] https://www.tylervigen.com/spurious correlations
[3] Hu, N., Pavlou, P. A., & Zhang, J. J. (2017). On Self-Selection Biases in Online
Product Reviews. MIS Quarterly, 41(2), 449-471.
[4] Wu, L., Hitt, L., & Lou, B. (2020). Data analytics, innovation, and firm productivity.
Management Science, 66(5), 2017-2039.