On November 2, the Advanced Macro Workshop on Machine Learning and Heterogeneous-Agent Models was held at Peking University HSBC Business School (PHBS), co-hosted by the Sargent Institute of Quantitative Economics and Finance (SIQEF) at PHBS, Institute for Advanced Study (IAS) and Economics and Management School at Wuhan University, as well as the University of International Business and Economics. During the event, more than 30 scholars from Chinese mainland and Hong Kong participated in discussions on the latest academic research in macroeconomics, machine learning, mechanism design, and heterogeneous-agent models.
Scholars and students attend the workshop
Nie Jun from Wuhan University
Professor Nie Jun gave a brief introduction to the workshop, highlighting its significance in bringing together researchers and experts from diverse institutions across mainland China and Hong Kong to discuss topics in machine learning, mechanism design, and heterogeneous agent models. In addition, the workshop also aimed to foster connections and collaborative learning between professionals in mathematics, data science, and economics.
Thomas J. Sargent, the 2011 Nobel laureate in economics and honorary director of SIQEF
Professor Thomas Sargent introduced the current research status and challenges surrounding the framework of decision problems known as "equilibrium Markov processes." He emphasized that, despite the conceptual simplicity, this high-dimensional structure poses significant challenges in fully characterizing it. However, with recent advancements in statistics, function approximation, and computing power, researchers are now better equipped to delve into the realms of machine learning and artificial intelligence. Professor Sargent expressed his hope that the presentations at the workshop would showcase innovative applications of these breakthroughs.
Lv Qi
Professor Lv Qi from Sichuan University presented his coauthored paper titled "Optimal Control of Stochastic Evolution Equations: Some Recent Progresses," discussing advancements in control theory for Stochastic Distributed Parameter Systems (SDPSs). Since existing methods for deterministic distributed parameter control systems and stochastic finite-dimensional control systems do not apply to SDPSs, it is necessary to develop new mathematical tools even for simple SDPSs. The paper mainly focuses on the controllability of stochastic hyperbolic equations and the Pontryagin-type maximum principle for controlled stochastic evolution equations, concluding with open questions and future research directions in SDPS control theory.
Mathieu Laurière
Mathieu Laurière, assistant professor at New York University Shanghai, delivered a speech on his collaborative paper, “A Machine Learning Method for Stackelberg Mean Field Games.” He introduced an innovative single-layer numerical method designed to tackle the Stackelberg mean field game problem. This method utilizes a penalty approach to convert the two-level problem involving the leader and the agents into a single-layer mean field optimal control problem. The findings demonstrate that the proposed reconstruction method converges to the original problem. Additionally, the research proposes a machine learning method utilizing feed-forward and recurrent neural networks and showcase its application through various examples from existing literature.
Jentzen Arnulf
Jentzen Arnulf, professor at the Chinese University of Hong Kong (Shenzhen) and University of Münster, presented his collaborative paper titled “Overcoming the Curse of Dimensionality: From Nonlinear Monte Carlo to the Training of Neural Networks”. This paper proposes an efficient machine learning algorithm for approximating solutions to high-dimensional partial differential equations (PDEs). The authors pointed out that deep artificial neural networks (ANNs) could effectively overcome the curse of dimensionality, making them suitable for a broad class of semilinear parabolic PDEs. Furthermore, the research findings indicate that certain smooth functions cannot be approximated by shallow ANNs without succumbing to the curse of dimensionality; however, they can be approximated by deep ANNs without this limitation.
Zhu Shenghao
Zhu Shenghao, the professor from University of International Business and Economics, presented a paper titled “Kantorovich Meets Sargent: A New Perspective of Rational Expectations". This paper explores an innovative approach to studying rational expectations within macroeconomic models, to examine how distributions or beliefs evolve theoretically and leverage machine learning to calculate these abstract constructs in macroeconomics. The author established a link between optimal transport theory, specifically through the Kolmogorov-Fokker-Planck equation, and rational expectations. This linkage offered a robust framework to trace the evolution of the density function of expectations within a heterogeneous agent model. By integrating machine learning techniques, the model elucidated intricate distributions of economic agents' beliefs and actions, thereby refining the accuracy of depicting belief evolution in dynamic economic environments.
Tang Qing
Tang Qing, a lecturer from China University of Geosciences, presented a paper titled "Cournot Mean Field Game of Controls: Theory and Numerical Algorithms." In this paper, Tang explored a sophisticated economic model where multiple agents optimize their production decisions in a Cournot competition setting. The model is particularly relevant for industries with finite resources, such as oil or other exhaustible commodities, where individual agents are influenced by the collective output of all players, thereby forming a Cournot Mean Field Game (MFG) framework. This framework integrates optimal control theory with mean field games, allowing agents to adapt their production strategies over time in response to fluctuating market prices and resource scarcity.
Chen Nan
Professor Chen Nan from the Department of Systems Engineering and Engineering Management of The Chinese University of Hong Kong shares his paper, “Collusion or Compete: A Two Timescale Evolutionary Game Approach to Algorithmic Collusion Study.” This paper solves the multi-agent reinforcement learning problems by replacing the Q-value function with the Nash Q-value function. To maintain stability, the authors slowed down the learning of policy function and belief function, and accelerated the learning of value function, therefore relaxing some restrictive assumptions for equilibrium and provide possibility for agents to reach the Nash equilibria in more complex cases. This research helps to understand algorithmic collusion of agents.
Zhang Lichen
Assistant Professor Zhang Lichen from Business School of the University of Hong Kong introduced the paper titled "College Access and Intergenerational Mobility." The authors combined the Aiyagari lifecycle model and the overlapping generations’ model to analyze parents' investment decisions in their children's education. The parameters of the model were determined through a two-step estimation process based on data from the China Family Panel Studies (CFPS). The paper found that an expansion of college capacity leads to a widening gap between high-income and low-income households, as high-resource households continue to have an advantage in investing in their children's human capital. Additionally, the paper revealed that education policies, such as increasing public education spending and reducing college costs, have only limited effects on improving intergenerational mobility in China. It suggested that policymakers should take into account family responses to achieve the desired effects.
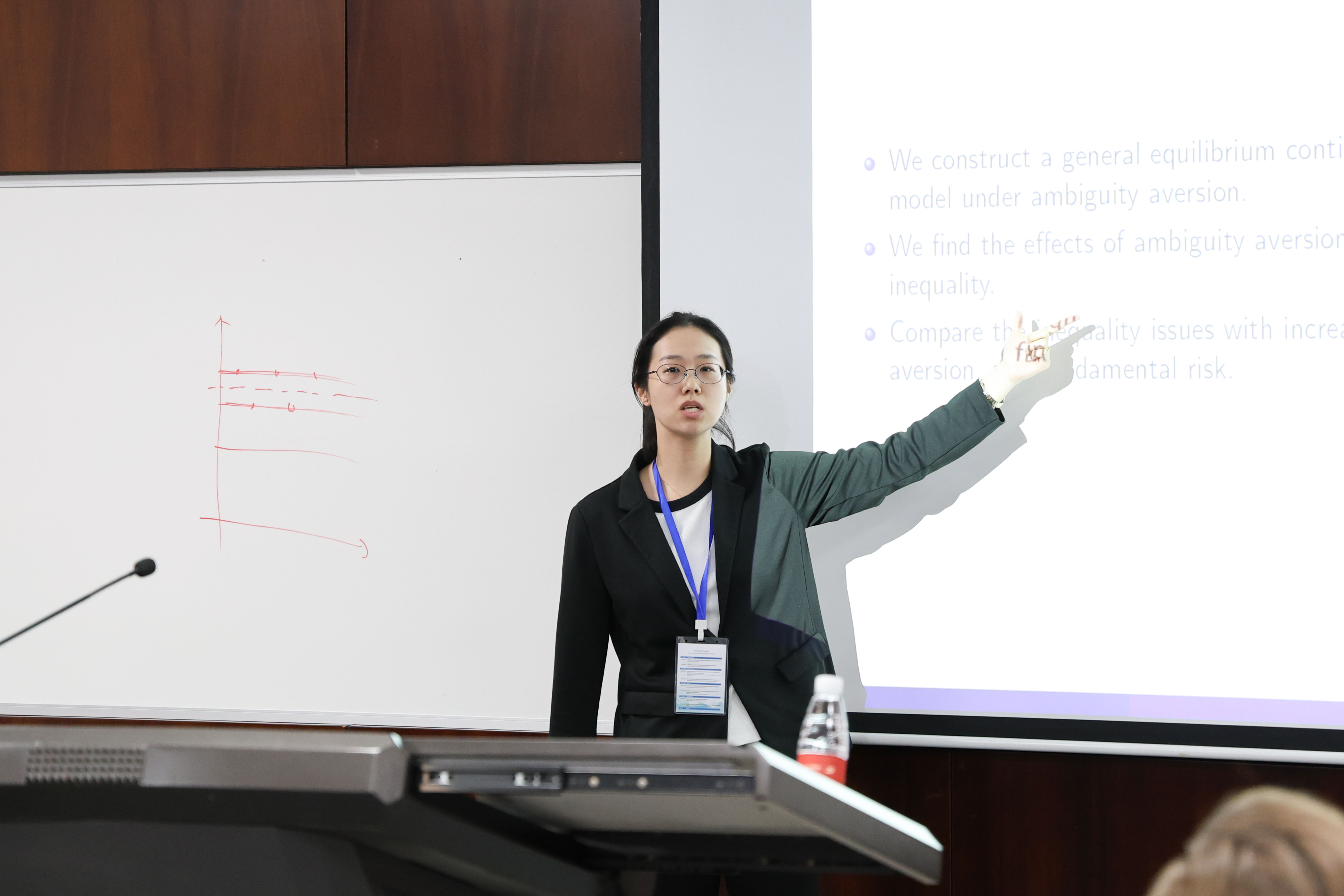
Fan Hua
Assistant Professor Fan Hua from the Institute for Advanced Economic Research at Dongbei University of Finance and Economics introduced the paper titled "Equilibrium Wealth and Consumption Inequality under Ambiguity Aversion." In this paper, the authors developed a continuous-time general equilibrium heterogeneous-agent model incorporating ambiguity aversion to investigate its impact on wealth and consumption inequality. They demonstrated that a higher degree of ambiguity aversion leads to lower inequality in both wealth and consumption, whereas higher risk aversion exacerbates such inequality. However, they also found that price effects from the general equilibrium may counteract or even reverse these outcomes. As aversion behavior can decrease demand and prices, higher ambiguity aversion may actually increase welfare.
The group photo of participants
This workshop not only provided an academic platform for researchers in related fields to exchange ideas but also injected new impetus into the development of machine learning and heterogeneous-agent models, fostering integrated development across interdisciplinary areas. It was supported by the Key Project of the National Natural Science Foundation of China, titled "Research on Machine Learning Algorithms and Applications for Heterogeneous Dynamic Macroeconomic Models" (72433004).
Source: Sargent Institute of Quantitative Economics and Finance, and Public Relations and Media Office
By Dong Xiao, Wang Weirong, Xiong Zichao, and Annie Jin